In the rapidly evolving landscape of software development, ensuring the quality and reliability of mobile applications has become paramount. As the complexity of apps continues to grow, so does the need for innovative solutions that streamline the testing process.
Artificial Intelligence (AI) is a competent technology poised to transform the field of application testing, potentially revolutionizing the entire industry.
This article will explore how AI test automation can effectively enhance the efficiency, accuracy, and overall effectiveness of app testing.
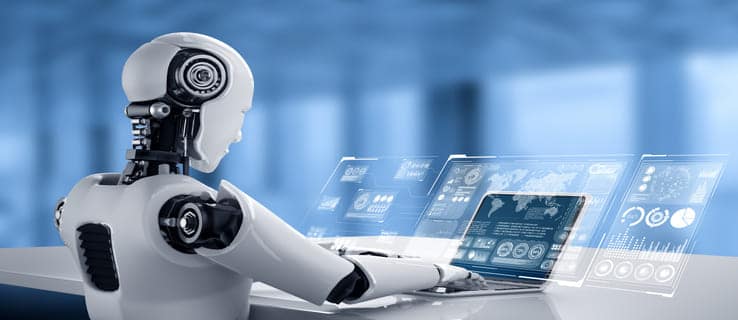
The Role of Artificial Intelligence in App Testing
AI has revolutionized the mobile application testing landscape, introducing advanced capabilities that streamline various testing tasks. Developers can automate repetitive and time-consuming testing procedures using AI techniques like ML and NLP (Natural Language Processing).
These intelligent systems can swiftly identify defects, analyze data, and generate insights to optimize performance.
By embracing the power of AI, developers and quality assurance teams can propel their testing methodologies to new heights, paving the way for exceptional app performance and user satisfaction.
Benefits of AI in App Testing
The integration of AI in app testing offers several noteworthy advantages, including:
Increased Efficiency
AI improves efficiency in app testing through automated test case generation, execution, and result analysis. By leveraging AI algorithms, testers can rapidly generate diverse test cases, reducing manual effort and saving time.
Enhanced Test Coverage
AI-powered techniques like machine learning enable comprehensive test coverage by analyzing vast data and identifying potential defects. Through intelligent pattern recognition, AI algorithms help detect hidden bugs and vulnerabilities that may go unnoticed in traditional testing methods.
Intelligent Test Prioritization
AI algorithms can prioritize test cases based on criticality, risk assessment, and usage patterns. By intelligently selecting the most relevant test scenarios, testers can focus on areas that are more likely to uncover high-impact issues, thus maximizing the effectiveness of testing.
Faster Bug Detection and Diagnosis
AI-powered tools can quickly analyze test results, identify anomalies, and pinpoint potential bugs or performance bottlenecks – this accelerates bug detection and diagnosis, enabling faster resolution and improved app quality.
Real-time Monitoring and Alerting
AI-based monitoring systems continuously analyze app behavior, performance metrics, and real-time user feedback. This proactive approach helps developers by detecting issues early, alerting them, and ensuring swift remediation – reducing the impact on end-users.
Test Data Generation and Management
AI techniques facilitate the creation and management of test data by intelligently generating realistic and diverse data sets; this helps ensure thorough testing across various scenarios and enhances the accuracy and relevance of test results.
Collaboration and Knowledge Sharing
AI-powered collaboration platforms enable seamless communication and knowledge sharing among testers, developers, and stakeholders. These platforms foster effective collaboration and facilitate faster decision-making by centralizing information and providing intelligent insights.
Implementation Strategies
Utilize AI in Test Case Generation
- Apply AI techniques to generate test cases based on system requirements automatically.
- Use machine learning algorithms to identify relevant test scenarios and optimize test coverage.
Employ AI-Powered Test Automation
- Leverage AI technologies to enhance test automation processes.
- Implement intelligent algorithms for test script creation, maintenance, and execution.
- Apply machine learning algorithms to detect patterns, anomalies, and potential defects in test results.
Incorporate AI in Test Data Management
- Utilize AI algorithms to generate test data.
- Apply data synthesis techniques to create representative datasets for testing.
- Leverage AI for data anonymization and protection to ensure privacy regulation compliance.
Integrate AI for Defect Prediction and Analysis
- Utilize machine learning models to predict potential defects based on historical data.
- Implement anomaly detection algorithms to identify abnormal behavior in applications.
- Leverage AI techniques for root cause analysis and efficient debugging.
Leverage AI in Performance Testing
- Apply AI algorithms to identify bottlenecks.
- Utilize machine learning models to predict system behavior under different loads.
- Leverage AI-based optimization techniques to enhance application performance.
Utilize AI for Test Environment Management
- Apply AI algorithms for efficient provisioning and configuration of test environments.
- Utilize machine learning models to predict resource requirements and optimize resource allocation.
Apply AI in Test Result Analysis and Reporting
- Leverage AI techniques to analyze test results and identify trends and patterns.
- Utilize natural language processing algorithms for automated report generation.
- Apply data visualization techniques to present test results effectively.
Remember to adhere to these implementation strategies when incorporating Artificial Intelligence in application testing.
Conclusion
By embracing AI in app testing, organizations can optimize their testing efforts, improve app quality, and drive customer satisfaction. The future of app testing lies in the effective utilization of AI technologies, and those who embrace this transformation stand to gain a competitive edge in the fast-paced world of software development.
AI is a game-changer in app testing, propelling organizations towards enhanced efficiency, greater accuracy, and improved app quality. Embracing AI-driven strategies paves the way for successful app releases, ensuring users enjoy reliable, high-performing applications in today’s dynamic digital landscape.